CVEDIA Synthetic Data is the Missing Link for AI
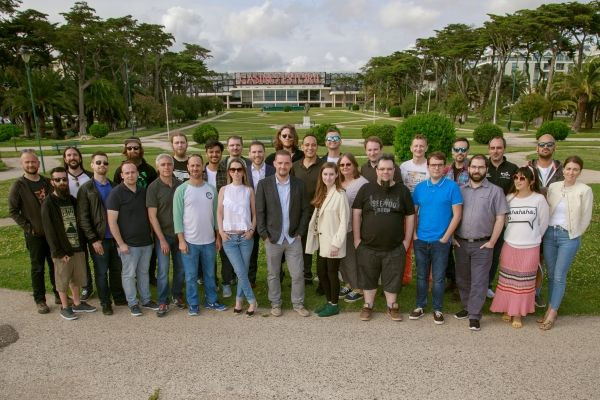
Artificial intelligence, or AI, is doing more than ever. From being deployed in health care and warfare, to helping people make music and books, the capabilities of AI are seemingly endless, and while AI has been around for some time now, roadblocks still exist in its development. In particular, data, necessary for the development of AI, is prohibitively costly in both terms of monetary value and time to acquire.
Imagine: a logistics and shipping company is developing AI to help ships navigate treacherous seas. Countless hours and sums of money are spent creating real-world scenarios of collisions and possible environmental anomalies to collect data needed to train an AI. This is not only costly but also time-consuming and potentially dangerous. However, this data is essential to an AI’s learning, especially where safety is concerned, so it needs to be accurate and realistic, and there’s no other way to collect this data than by creating real-world scenarios and collecting the data from that - or is there?
This missing link lies in synthetic data. Essentially, synthetic datasets are artificially manufactured using big data and machine learning theory instead of a real-world collection. This data is anonymized and created based on user-specified parameters, and it’s recently proved to create the same results as real-world data, provided the developers are able to accurately simulate real-world conditions.
A major player at the center of this solution is CVEDIA, or Computer Vision Encyclopedia, known for their development of the first SaaS AI platform that provides users with advanced image and video processing tools to clean noisy datasets for computer vision projects. Since this development, CVEDIA has turned their sights to “synthetic data” and how it can solve real-world AI projects through more realistic testing.
“We create state-of-the-art synthetic datasets for algorithm training.” Says Arjan Wijnveen, CEO at CVEDIA, “these datasets are designed to be entropic and concentrated, with unexpected scenarios, lighting and condition edge cases, system failure possibilities and other anomalies. Essentially, we focus on addressing specific challenges data scientists face when training AI to detect, monitor, and react to situations safely and correctly by ensuring a large amount of variety and a reduction of gaps in our synthetic `datasets.”
In the context of a shipping company using AI to help ships navigate treacherous seas, this is especially valuable, as many worst - and best-case scenarios can be explored without even entering the ocean. Synthetic data for almost any type of real-world event can be created, without having to face the costs or potential danger of real-world data collection.
“Physical data-collection sensors and equipment are expensive, that, combined with the costs and logistics of real-world testing pushes the budget out of scope for many companies,” says Wijnveen. “You can’t realistically launch a boat and purposefully subject it to high seas, powerful storms, or collisions for the purpose of data-gathering.”
This may raise some potential concerns with safety - after all, if the AI hasn’t been exposed to realistic conditions, how can it realistically react when it inevitably faces a real-world issue?
“We have optimized our synthetic data by partnering with leading sensor producers to compare synthetic datasets against legitimate data.” says Wijnveen “In tests comparing the performance between the two, the synthetic tests have - at times - outperformed the real-world data.”
Other applications for this synthetic data are essentially limitless - from making cities safer and smarter, to detecting strawberries at each stage of their growth cycle, CVEDIA has the capability to produce synthetic data for almost any scenario:
“AI solutions are fundamental to the safety of our cities,” says Wijnveen, “AI has the ability not only to increase the safety of dangerous intersections but also in the training of machine learning systems. Through our synthetic data, clients are able to identify and understand dangerous or at-risk areas of cities, enabling them to create effective systems to keep their citizens safe.”
CVEDIA’s synthetic data for smart city design enables developers to fill in data gaps for scenarios that are prohibitively expensive or unacceptable to reproduce in real life - like traffic accidents or traffic jams. Synthetic data also helps to avoid the privacy and regulatory issues that arise when data is collected from existing, physical devices, such as red light or other traffic cameras. Through creating these effective datasets, synthetic data enables AI to recognize people, vehicles, bicycles and city infrastructure, and through machine learning, find and implement solutions to make cities safer and more efficient.
On the future of AI, Wijnveen states, “While it still faces some challenges, CVEDIA is well on the way to speeding up and acting as the missing link to AI’s development through synthetic data - creating an essential tool to supplement existing data and augment machine learning algorithms to solve countless problems.”