The Power of Small: How SLMs Are Improving AI Safeguards, According To Aporia
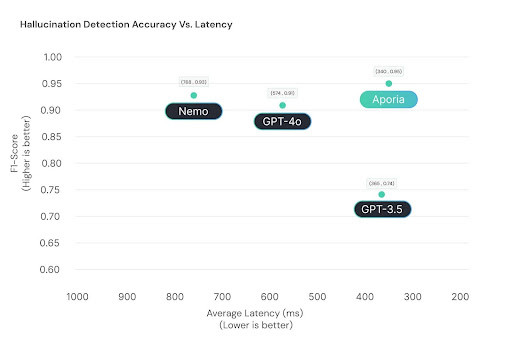
If the quick rise of artificial intelligence (AI) has taught the world anything, it is that development and risk often go hand in hand. As AI tools become ubiquitous in everyone's daily lives, offering remarkable convenience and capabilities, they also bring many concerns.
The need for robust oversight of these powerful technologies has never been more urgent, from the spread of misinformation to privacy breaches and even tragic incidents linked to AI interactions. AI control platforms like Aporia are addressing these challenges.
The concept of AI safeguards has become crucial in guaranteeing the responsible deployment of AI technologies. With the implementation of the European Union Artificial Intelligence Act and similar regulations worldwide, there is growing recognition that AI systems cannot operate unchecked.
What is an SLM?
Small language models (SLMs) represent a notable change in AI, offering a compelling alternative to their larger counterparts. While large language models (LLMs) like GPT-4 have captured the public imagination with their vast capabilities, SLMs are establishing a niche that prioritizes efficiency and specialization.
An SLM is a compact version of an LLM designed to operate with significantly fewer parameters. LLMs might boast billions or even trillions of parameters, while SLMs may contain only a few million.
This reduction in scale, however, does not necessarily translate to a proportional decrease in capability. Instead, developers optimize SLMs for specific tasks, allowing them to perform remarkably efficiently in their designated domains.
The primary advantage of SLMs lies in their fine-tuning ability for particular applications. This enables them to perform well in targeted areas, often rivaling or surpassing larger models in specific tasks. Their smaller size also reduces computational requirements, faster processing times, and lower operational costs.
SLMs vs. LLMs: Comparing the Two
LLMs have garnered attention due to their broad capabilities and human-like text generation, but SLMs offer distinct advantages that make them particularly suitable for certain applications, especially in AI safeguards.
LLMs excel in tasks requiring broad knowledge and complex reasoning. They can generate human-like text across various topics and adapt to various contexts. However, this versatility comes at a cost. LLMs are resource-intensive, requiring substantial computational power and memory. They also face challenges with latency, especially as the input size increases.
Developers design SLMs for efficiency and specialization. While they may not match the breadth of LLMs, engineers can fine-tune them to perform specific tasks with high accuracy. This specialization makes them particularly effective for applications like content moderation, sentiment analysis, or specific types of text classification—tasks crucial for AI safeguards.
One of the most significant drawbacks of LLMs in the context of safeguards is their struggle with multitasking. As the complexity of the task increases — for instance, simultaneously checking for fabrications, prompt injections, personally identifiable information (PII), and toxicity—LLMs tend to lose accuracy. This limitation is analogous to asking a child to perform multiple complex tasks at once: the likelihood of error increases substantially.
Furthermore, LLMs face challenges with latency that become more pronounced as the input size grows. A study by Glean showed a linear relationship between the number of input tokens and the time to first token (TTFT). This correlation affects response speed and potentially impacts accuracy, as the model may struggle to process all the information effectively.
The multiSLM Architecture
Aporia's inventive multiSLM architecture indicates a fundamental change in AI safeguards. This system uses multiple specialized SLMs, each fine-tuned for a specific task or policy enforcement. The architecture addresses the limitations of traditional LLM-based approaches while maximizing efficiency and accuracy.
In the multiSLM system, incoming queries are distributed across various specialized SLMs. For example, one SLM might detect prompt injections, another identify potential fabrications, and another recognize toxic content. This division of labor allows for parallel processing, significantly reducing overall latency.
The effectiveness of this architecture lies in its modularity. Developers optimize each SLM for a particular task, allowing it to perform accurately and quickly, enabling the system to handle complex, multi-faceted queries that would typically challenge an LLM-based method.
Moreover, the multiSLM architecture is inherently adaptable. As new challenges or requirements emerge, engineers can integrate additional specialized SLMs into the system without disrupting the existing structure. This flexibility guarantees that the safeguards can progress alongside the rapidly changing AI field.
Advantages of the multiSLM Architecture for Safeguards
The multiSLM architecture offers several compelling advantages that make it particularly well-suited for implementing effective AI safeguards:
Exceptional Speed: By distributing tasks across multiple specialized SLMs, the system can process queries at remarkable speeds. Each SLM, being smaller and focused on a specific task, can operate with minimal latency. The parallel processing capability allows multiple checks to be performed simultaneously, resulting in overall response times often measured in milliseconds rather than seconds.
Improved Accuracy: Fine-tuned SLMs have demonstrated superior performance in specific tasks compared to larger, more general models. This specialization allows each SLM to achieve high accuracy in its designated role, whether it detects fabrications, identifies prompt injections, or recognizes inappropriate content. The cumulative effect is a safeguard system that offers precise and reliable protection across multiple dimensions.
Economical: The use of smaller, more efficient models translates to reduced computational requirements. This lowers operational costs and makes advanced AI safeguards accessible to various organizations, from startups to large enterprises.
Adaptability and Flexibility: The multiSLM architecture's modular nature allows for easy expansion and adaptation. Engineers can add new SLMs to address emerging challenges or comply with new regulations without overhauling the system.
Reduced Fabrications: By using specialized models for specific tasks, the multiSLM approach significantly reduces the risk of fabrications—a common problem with larger, more general models when faced with complex or ambiguous queries.
Aporia's multiSLM Detection Engine
Aporia's multiSLM detection engine represents the practical implementation of this inventive architecture. Designed to protect any generative AI (GenAI) application in real time, it offers a wide range of safeguards against various security and reliability threats.
The system allows developers to select and configure specific safeguard policies designed to their AI application's needs. These policies can address particular concerns, such as preventing prompt injections, mitigating fabrications, or filtering out inappropriate content..
One of the key features of Aporia's engine is its ability to act immediately. When a message violates a policy, the system can immediately intervene, either by blocking the content, rephrasing it, or taking another predefined action. This real-time capability is crucial for preventing potential harm before it occurs.
Aporia's system also offers extensive monitoring and analytics capabilities. A live dashboard provides real-time updates on user and AI interactions, flagging policy violations and the actions taken. This level of transparency and control allows organizations to maintain a clear overview of their AI system's behavior and make data-driven decisions to improve safety and reliability.
SLMs Are The Future of AI Observability
The advent of small language models and inventive architectures like Aporia's multiSLM truly system marks a notable milestone in the quest for effective AI safeguards. It offers a compelling solution to the challenges posed by traditional LLM-based safeguards by harnessing the power of specialization and parallel processing. The result? Companies finally have a much-needed framework for AI safety that is more apt to the progressing space of AI technologies and regulations.
As organizations deal with the dual challenges of harnessing AI's potential while guaranteeing its responsible use, solutions like Aporia's multiSLM detection engine are set to have a massive role, balancing progress with safety, efficiency, and reliability.
© Copyright 2025 IBTimes AU. All rights reserved.